The utility and limitations of large-scale surveys during the COVID-19 pandemic
Large-scale surveys for public health, with a focus on COVID-19
Large-scale surveys can collect health behavior data from people in diverse geographic settings. Survey data can estimate population-level behaviors such as smoking, health care access, or adherence to cancer screening, and the results of data analysis can guide public health authorities as they monitor progress and take actions to improve health. Although self-report surveys have well-recognized limitations, the advantages they offer, including lower costs and the potential to reach large numbers of people, can make them a valuable asset in many circumstances.
Some of the data used to define global and national public health agendas come from large-scale surveys. Examples include the World Health Survey conducted by the World Health Organization through interviews, or the Behavioral Risk Factor Surveillance Survey conducted by the U.S. Centers for Disease Control and Prevention (CDC) by telephone. Self-report data can be collected through surveys conducted by telephone or mail-in questionnaires, in-person interviews, and increasingly, through internet-based platforms that can deploy a standard survey to large numbers of respondents.
During the COVID-19 pandemic, lack of complete and timely data has hindered the response. While public health data systems adapted existing infrastructure to quickly record and share information about measures such as cases, hospitalizations and deaths, other types of information have been more difficult to obtain. This is especially true for real-time data on changes in human behavior as the pandemic, recommendations on mitigation measures and the availability of vaccines evolve. Examples of information that is critical to guide the pandemic response include adherence to public health and social measures such as mask wearing and social distancing, readiness to receive a COVID-19 vaccine, data on symptoms that may be underreported but may serve as an early warning of disease spread, and the impacts of the pandemic and response on individual health. Some states, such as Utah, have added questions about behavioral factors related to COVID-19 to their Behavioral Risk Factor Surveillance Survey as a way to collect some of this information. However, telephone surveys are labor intensive, time consuming and expensive. Furthermore, decreases in response rates to telephone surveys can both increase costs and reduce representativeness. Given the number of people that can be reached quickly and at low cost through internet-based platforms, digital collection tools have been developed around the world to fill information gaps that can help guide the COVID-19 response. Some of these tools are designed to gather scalable longitudinal or cross-sectional data on population-level patterns of important measures beyond cases, hospitalizations and deaths.
Several large-scale surveys have launched over the past year to assess aspects of the COVID-19 pandemic. The COVID States Project launched periodic national surveys to collect information on health behavior and adherence to physical distancing, mask wearing, and hand-washing, among other risk reduction behaviors. Globally, a number of surveys deployed through smartphone applications have been used to collect similar information. One is the application-based platform from the COVID Symptom Study, built in the United States to support the Nurses’ Health Study and now used to collect information about COVID-19 symptoms in the U.S., U.K. and Sweden. Another large-scale COVID-19 global data collection platform, the COVID-19 World Symptom Survey, is implemented by Facebook. Data collected via Facebook in the U.S. and other countries are freely available to the public and are thus a useful source of information on symptoms and behavioral trends during the pandemic.
Potential applications of large-scale self-reported symptoms
Data from the COVID Symptom Study have been used to show that anosmia, or loss of smell, appears to be a strong predictor for COVID-19. In addition, although fever alone is not particularly discriminatory for COVID-19, among those with fever in combination with less common symptoms such as vomiting or diarrhea, a greater frequency of positive tests have been observed. In Wales, users of the COVID Symptom Study app reported symptoms that predicted, five to seven days in advance, two spikes in the number of confirmed COVID-19 cases; a decline in reports of symptoms preceded a drop in confirmed cases by several days. Data from the COVID Symptom Study have also been used to support empirical research on smoking and COVID-19 risk; among more than 2.4 million survey respondents, current smokers were more likely to report symptoms suggestive of COVID-19. In another analysis of the COVID Symptom Study researchers analyzed data submitted by 2.8 million users in the U.K. during March-September 2020, with 120 million daily symptom reports and the self-reported results of 170,000 PCR tests to estimate the incidence of infections with SARS-CoV-2, the virus that causes COVID-19. There was a high degree of correlation between those estimates and confirmed case counts in large national COVID-19 datasets.
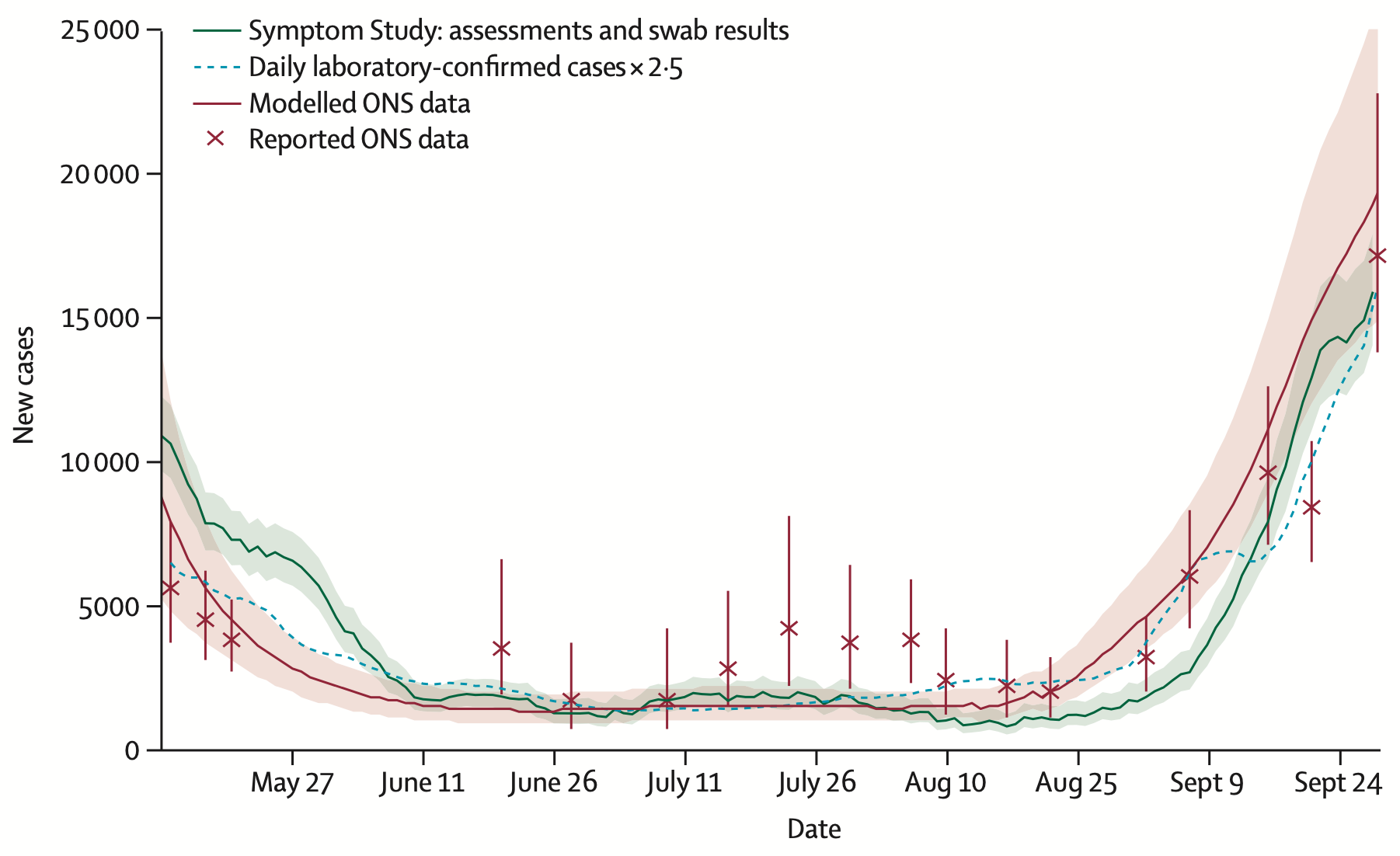
In that same analysis, researchers also used COVID Symptom Study data to successfully identify 15 of the 20 regions in England that, according to government data, had the highest incidence of COVID-19 in September 2020. Results suggest that self-reported symptom data may be used to 1) accurately estimate COVID-19 incidence and 2) forecast incidence in regions with low rates of testing or when test result reporting is delayed.
In the U.S., surveillance for COVID-like illness is conducted nationally and data are tracked by the CDC. These data on health care visits for people with symptoms that may be due to COVID-19 can also serve as an important early warning of COVID-19 spread.
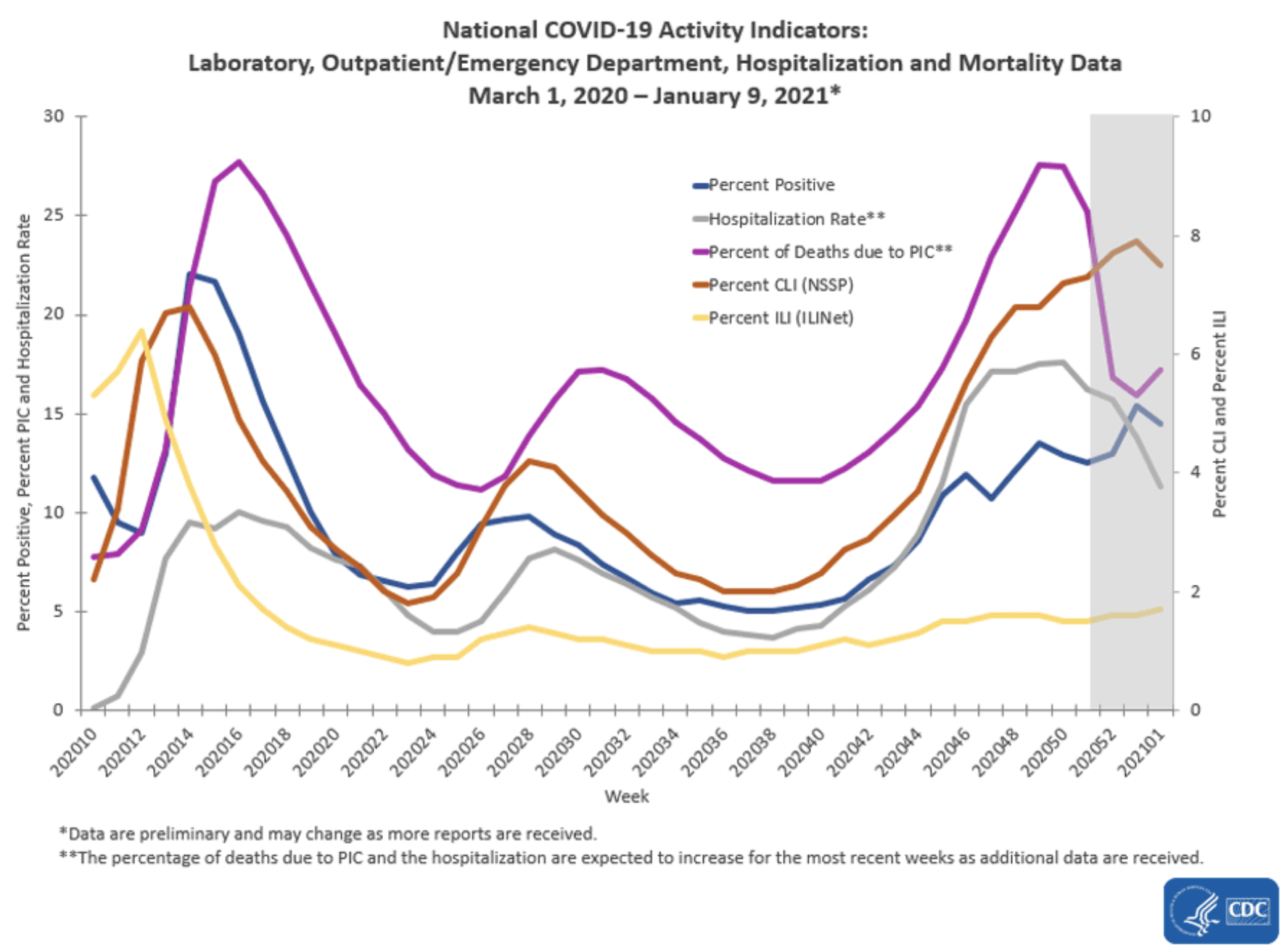
Large-scale surveys may have advantages over traditional health care facility-based syndromic surveillance. Not everyone with symptoms seeks care, and COVID-like illness data collected from patients receiving care at healthcare facilities may underreport the prevalence of symptoms in the community, especially among populations less likely to seek care. Frequent monitoring by daily reporting to an application may capture signals more rapidly than health care provider reporting systems. Drawbacks to the use of symptom data collected through an internet-based platform include missing data from people who may be at greater risk of COVID-19 yet are not internet savvy or not on Facebook (e.g. people who are elderly or live in long-term care facilities). In addition, people may be more likely to misrepresent their symptoms when reporting to an application than when they are interviewed by a health care professional.
In Brazil, the global symptom survey data was able to identify a new wave of cases three weeks before detected by official government sources. Resolve to Save Lives and Vital Strategies conducted a validation analysis (currently under peer review) and found a high correlation of self-reported symptom prevalence of COVID-like illness and the date of onset of severe acute respiratory illness cases, allowing for real-time monitoring of disease activity. Global symptom survey data might be most valuable where there are limitations in testing availability, access, or substantial delays in data reporting.
Self-report survey data gathered online may also be used to examine the association between mask use and disease transmission over time and between places. One preprint analysis showed that in U.S. states where a high percentage of people reported face mask use, there was a higher probability of controlling COVID-19 transmission. Serial cross-sectional surveys on likelihood of wearing a mask to the grocery store or with family and friends were administered in June-July 2020, via a web platform, to more than 350,000 people. Most (84.6%) reported being likely to wear a mask to the grocery store, while less than half (40.2%) reported being likely to do so when visiting friends or family. In a multivariate model adjusting for confounders including social distancing, a lower reported likelihood of mask wearing was associated with increased community transmission. A limitation is that it is difficult to disentangle people’s mask adherence from their adoption of other preventive measures. Another preprint analysis showed that mask use has changed differently across countries during the COVID-19 pandemic. Researchers used data from 13.7 million responses to a daily online survey completed by people in 38 countries between April and October 2020. In 13 countries, mask use stayed at 70% or higher throughout the study period, while in Denmark, Sweden and Norway, mask use consistently remained below 15%. In most other countries, mask use was low in April and eventually reached higher levels. Sociodemographic factors (e.g. older age, female gender, education, urban residence) and stricter mask-related policies were associated with higher mask use in public settings.
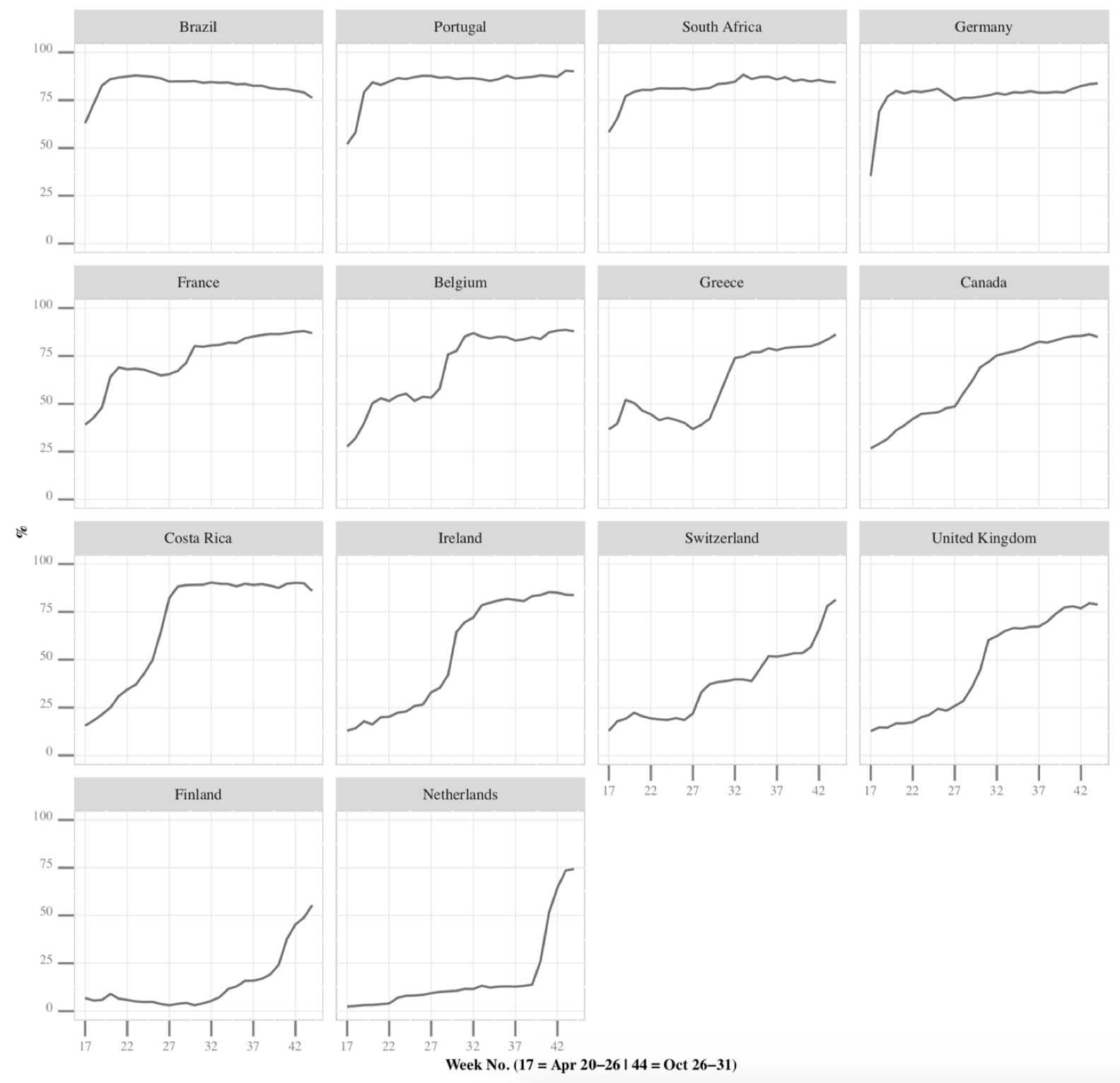
On the X-axis is the percentage of people who reported wearing masks in public; on the Y-axis is time in weeks. Week 17 began April 20, 2020, and Week 42 ended October 31, 2020. Source
Collection and management of large-scale self-reported internet-based survey data: COVID-19 World Symptom Survey data as a case study
The methods used to collect and manage data from large-scale self-reported internet-based surveys have implications for how results are interpreted and applied. In the case of the COVID-19 World Symptom Survey, participants are recruited on Facebook. The Delphi Research Group at Carnegie Mellon University designs and runs the surveys administered in the U.S., while researchers at the University of Maryland design and run the surveys administered in other countries. The surveys, which can be viewed online, include questions about symptoms that may be due to COVID-19 (e.g. fever, cough, loss of taste or smell). Additional questions, which vary between U.S. and global surveys, ask about demographics, mask-wearing and other behaviors, household financial concerns, and willingness to get a COVID-19 vaccine. The study population is active Facebook users who are at least 18 years old, live in any of more than 200 countries or territories and use one of more than 50 supported survey languages. Every day, Facebook invites a random sample of users, stratified by country, to take the survey via an invitation at the top of their news feed. Those who view the invitation are redirected to an off-Facebook site where the survey is administered. Partnering academic institutions receive the survey responses and send Facebook a list of random identification numbers that were assigned to survey respondents. Facebook uses internal data consisting of self-reported age, sex and geographical variables to weight results in accordance with population age and sex distributions in the respondents’ respective countries. In the U.S., results are available down to the county level. Data may be visualized on the COVIDcast map and survey overview statistics for the U.S. and on the COVID-19 World Survey Map for the rest of the world. In the U.S., since the survey launched in March 2020, an average of 250,000 people have submitted survey responses each day. Globally, over 30 million surveys have been completed. Facebook is also leveraged to recruit participants for the COVID-19 Beliefs, Behaviors and Norms survey, designed and run by researchers at the Massachusetts Institute of Technology. All of these surveys are solely opt-in, all results are anonymous, and no information on individual responses is available to Facebook. In the sections below, data collected in the U.S. using surveys designed by the Delphi Research Group will be referred to as data from Delphi surveys.
Potential limitations of self-reported survey data
Despite the benefits and attractiveness of collecting and using large-scale self-reported survey data during the COVID-19 pandemic, there are potential drawbacks and limitations including several types of bias, or systematic errors in how self-report data are collected, that may make study results less reliable.
Type of bias | What does it mean? | How can it be minimized? | Example relevant to the COVID-19 pandemic |
---|---|---|---|
Non-response bias | People who choose to respond to a survey may be different from people who choose not to respond. | Keep surveys short, simple and relevant, and try to get information about people who are completing the survey as well as those who are not. | People who don’t respond to the survey may be those who don’t wear masks. |
Recall bias | People cannot remember a distant event or behavior, or certain people (e.g. those affected by a disease) are more likely to remember the event. | Select short recall periods when asking about events in the past, and offer “explainer” prompts (such as a list of symptoms) to capture more accurate data. | People who know someone recently diagnosed with COVID-19 may be more likely to remember their symptoms and exposures. |
Social desirability bias | People choose to answer a survey question with the answer they think is ethically or morally correct rather than the truth. | Attempt to validate a survey instrument before using it for data collection, e.g. compare to observational data or correlate with another objective measure. | People may report wearing masks more often than they actually do if they think it is the correct thing to do. |
Selection/sampling bias | People who are invited to participate in the survey are not representative of a larger population. | Ensure that the survey is deployed in a manner that reaches a broad range of people. | People without internet access or people less likely to use mobile devices do not have access to app-based or web-based COVID-19 surveys. |
Large-scale self-report data for mask use and comparison with alternative data sources
An abundance of evidence shows that the widespread use of masks in the community decreases transmission of COVID-19. For this reason, large-scale mask use data may be essential to public health action planning during the pandemic. However, there are no national systems for monitoring mask use behavior in the U.S., outside of self-report surveys, and the accuracy of self-reported mask use data is unknown. Plus, as the table above shows, there are well-described and potentially problematic sources of bias when data are collected by self-report. It is important to think critically about these issues and compare self-reported data to existing alternative data sources, such as studies in which mask use has been directly observed.
Data from Delphi surveys show that a relatively high percentage of people in most U.S. states report using masks while in public.
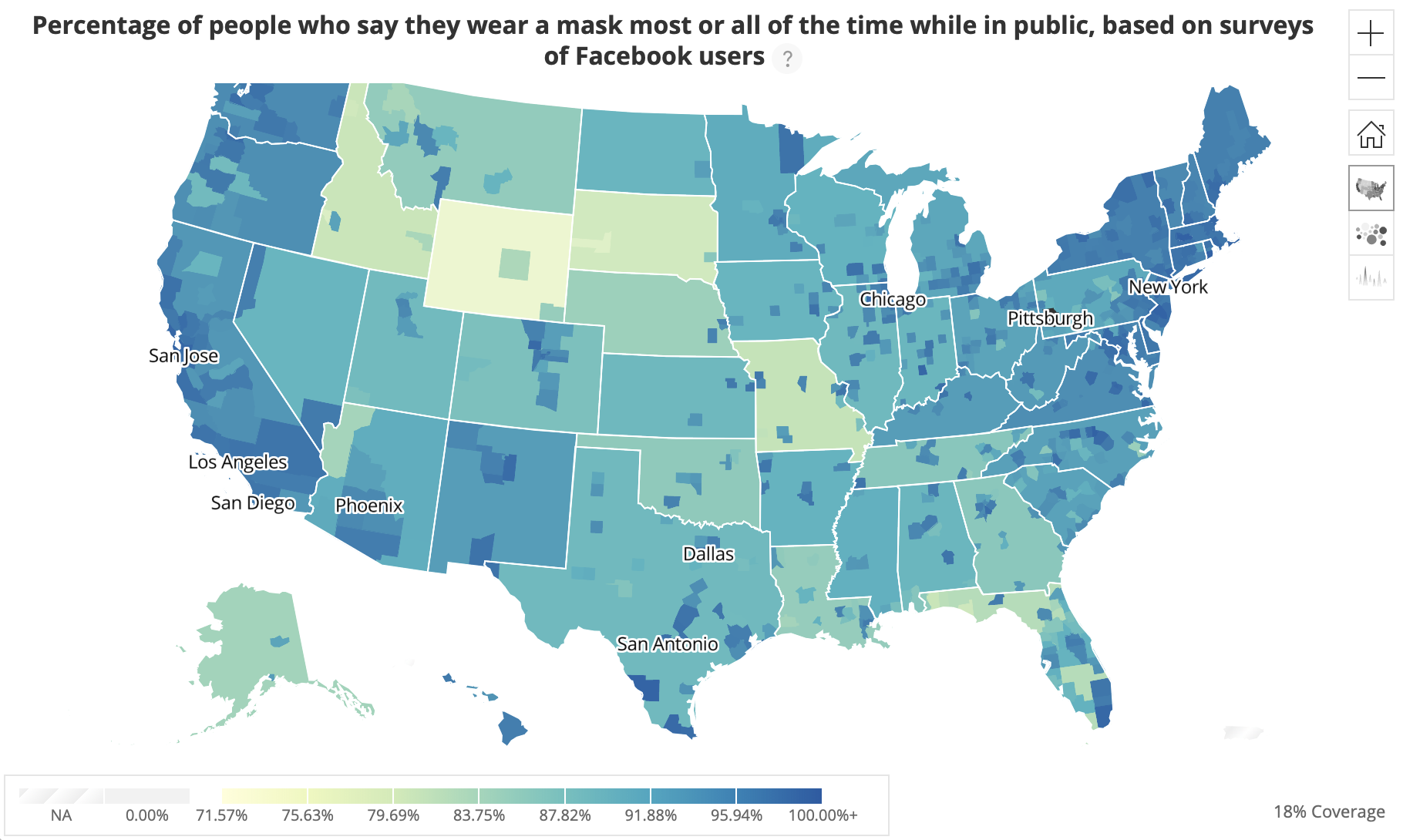
Generally, as shown in other studies, the percentage of people who report wearing masks in public has increased over time. On Jan. 12, 2021, approximately 94% of more than 250,000 Delphi survey respondents reported that during the past five days they wore a mask most or all of the time when in public.
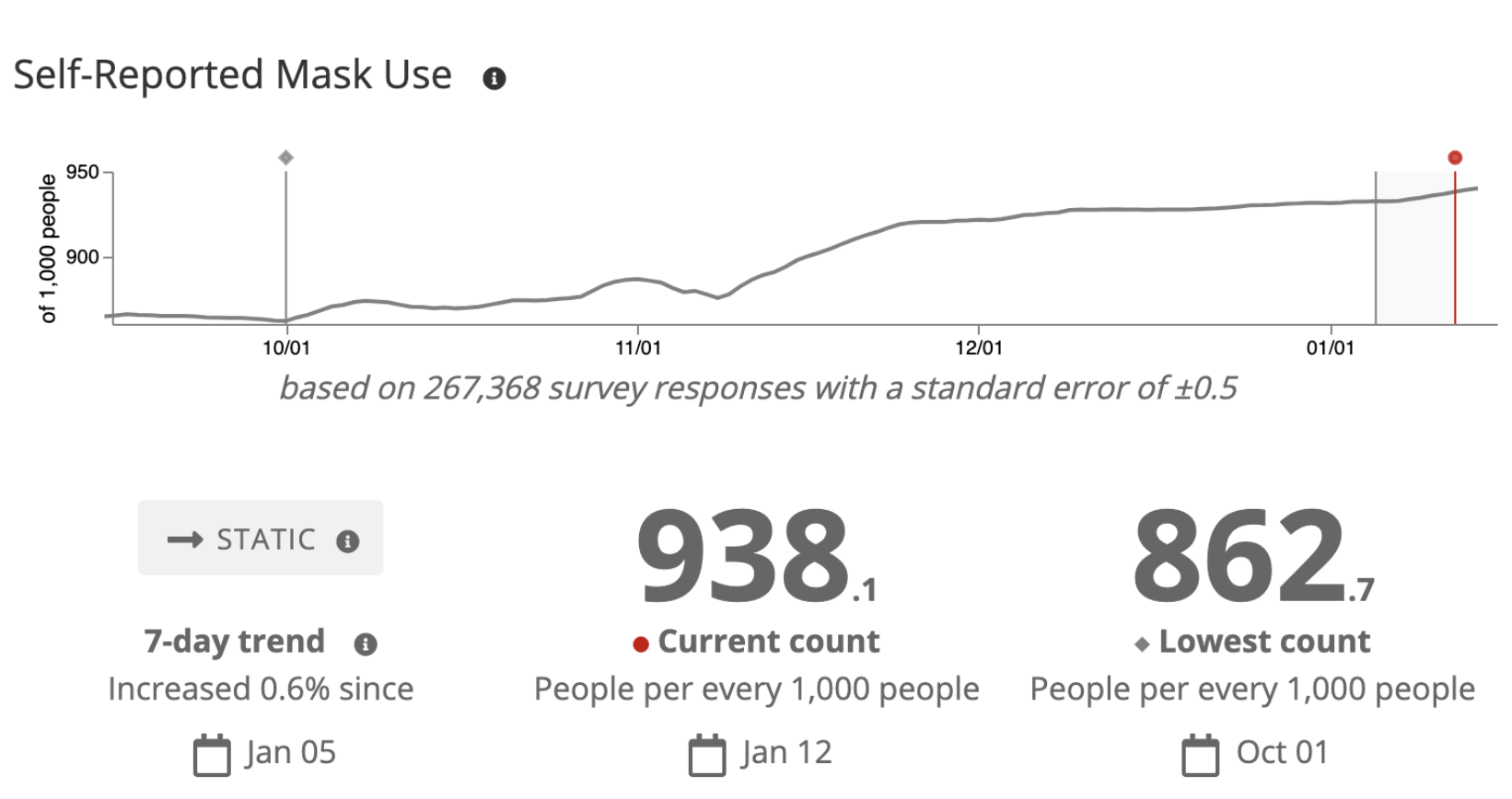
Self-reported rates of mask use may overestimate true rates of mask use adherence if survey respondents feel they should be wearing masks and answer accordingly. To attempt to circumvent this social desirability bias, Delphi surveys also include a question about whether others wear masks in public. These reported rates of mask use are slightly lower than reported rates among respondents themselves, but proportions are still high. On Jan. 12, approximately 82% of more than 250,000 respondents reported that during the previous seven days most or all other people wore a mask in public spaces where social distancing was not possible.
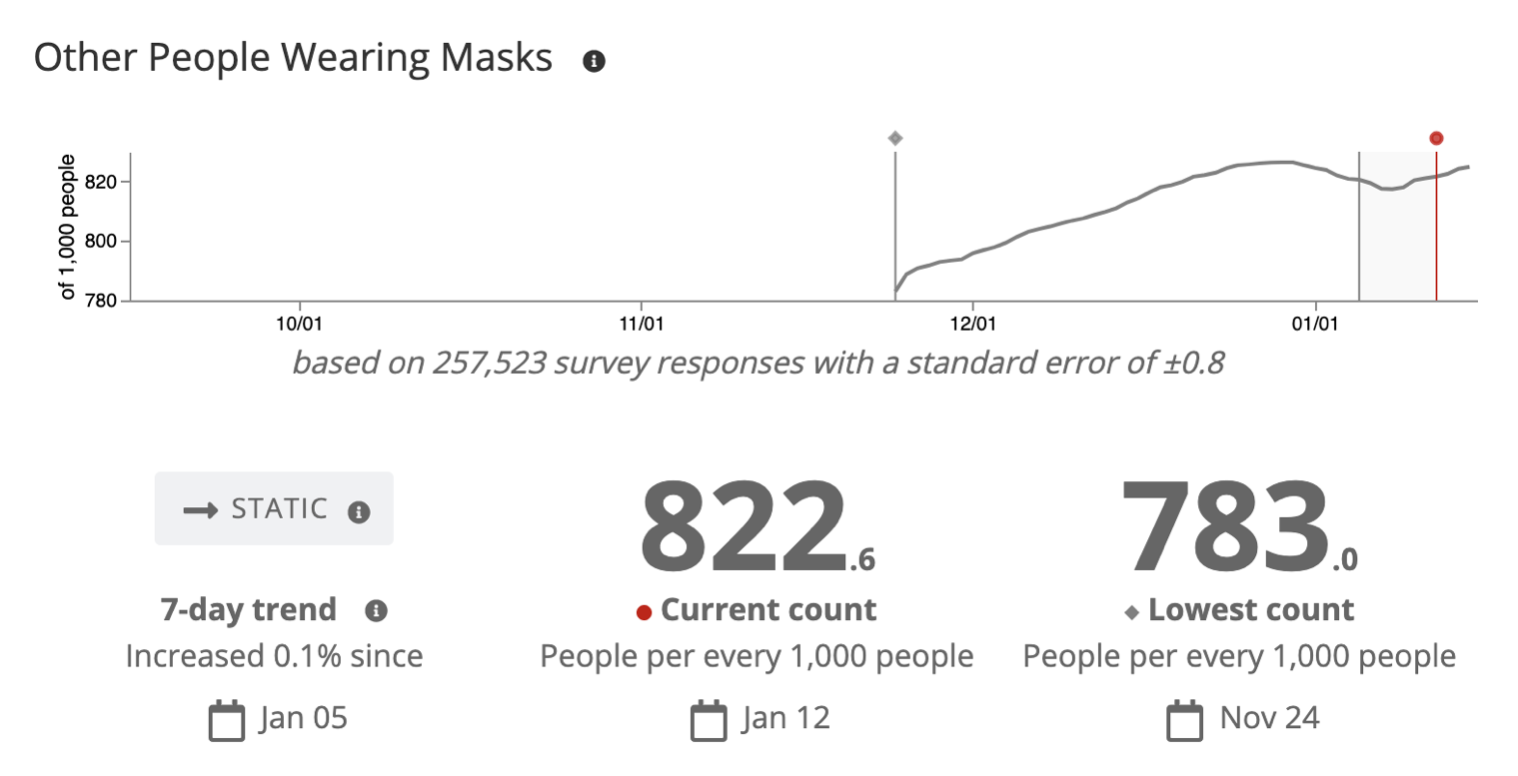
The accuracy of self-reported mask use data can be explored by comparing these data with directly observed mask use data from studies in which trained observers record the percentage of people they see wearing masks in selected observation locations. In-person observation or camera footage may be used to observe mask use. For example, the Philadelphia Department of Health collects data on mask use by observing security camera footage from approximately 50 cameras placed around Philadelphia in outdoor locations and just outside retail stores to approximate mask use patterns inside those stores. In the chart below, the results of the Delphi survey for Philadelphia County, from an average of more than 600 people per week, are compared with the results of direct observation of a similar number of people captured on camera footage each week.
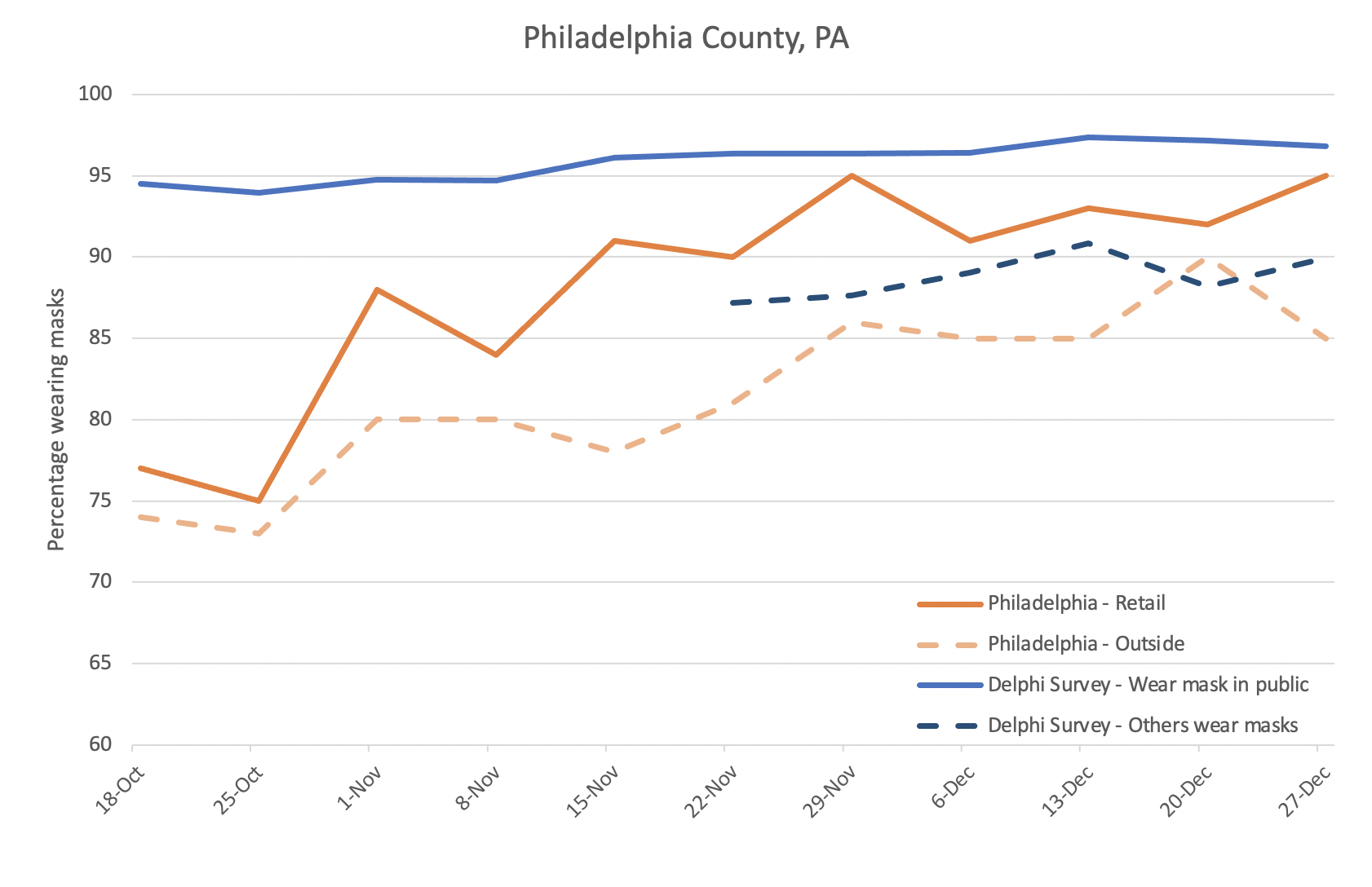
Data for the “Philadelphia” trend lines are direct observation data from the Philadelphia Department of Health; data for the “Delphi Survey” trend lines are from Delphi surveys accessed via Facebook.
It appears that estimates of population mask use all trend toward higher rates of mask use over time. Even the lowest estimates suggest that in late December more than 85% of people wore masks in public places. Data from Delphi surveys gathered via Facebook yield the highest estimates when people report on their own mask use; estimates from reports on others’ mask use fall between estimates of retail and outdoor mask use from directly observed camera footage. Of note, Philadelphia has had a mask mandate in effect since June 2020, which may influence mask use rates and also increase the effect of the social desirability bias. The above comparison suggests that estimates of mask use adherence from self-reported data approximate estimates of mask adherence from directly observed data, but that self-reported personal mask use may exaggerate the prevalence of mask use.
Another public health department that reports on mask use adherence is the Utah Department of Health. Data on mask use adherence is reported from two different sources. First, observers record mask compliance in a convenience sample of public spaces. The vast majority of these data come from indoor locations. Second, Utah collects self-reported data on mask use from the state Behavioral Risk Factor Surveillance System, a random-digit dial telephone survey of adults aged 18 and older. This survey asks how often the respondent wears a mask in public or when unable to socially distance. In the chart below, three types of data are presented: direct observations of an average of 365 people (range 24 to 1,041) per week, telephone surveying of an average of 186 people (range 140 to 231) per week, and self-reported mask use from an average of more than 2,500 people per week.
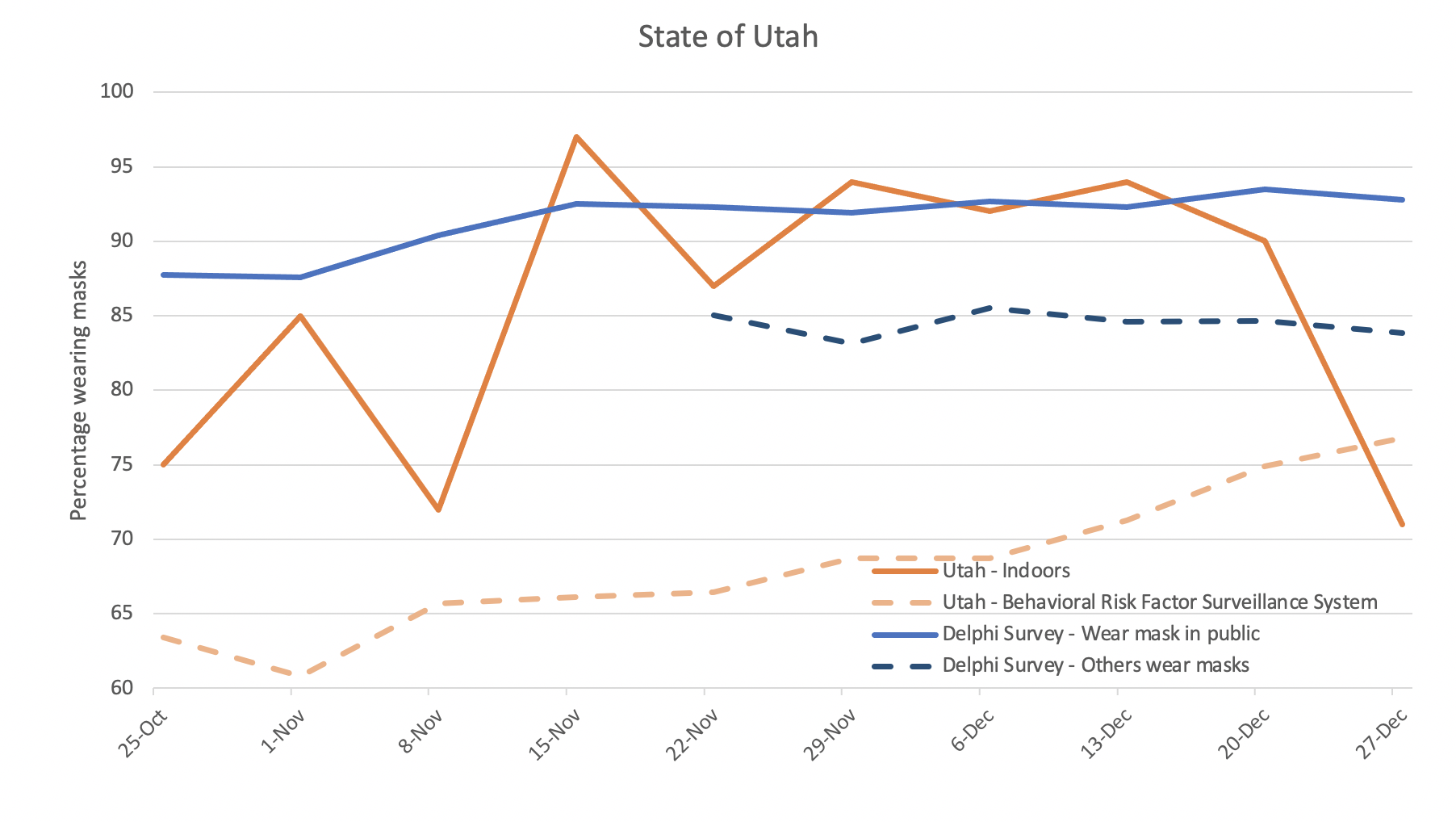
Data for the “Utah” trend lines are direct observation data from the Utah Department of Health and telephone survey data from the Behavioral Risk Factor Surveillance System; data for the “Delphi” trend lines are from Delphi surveys accessed via Facebook.
There is less correlation between mask use data from different surveys administered in Utah than there is between surveys administered in Philadelphia. Several factors may explain this. For one, the observational sample size in some weeks was quite low which may explain the large changes in estimated percentages from week to week. The convenience sample of locations in which direct observations were conducted implies that the population sampled may have changed significantly week to week. This is in contrast with data from Delphi surveys gathered via Facebook from thousands of respondents each week—these data may have been biased toward a certain segment of the population, but that bias was likely relatively similar over time. The above comparison illustrates the importance of understanding how sampling bias may impact survey results and that large-scale data are more robust to changes in the effects of sampling bias over time.
Delphi survey data do not reveal if respondents were wearing masks correctly, as can be measured through direct observation. For example, in a study conducted by the CDC and West Virginia University, of 3,144 people observed during Oct-Nov 2020, 2,637 (84%) wore masks and 2,269 (72%) did so correctly. Nor do Delphi data describe whether respondents wear masks in all settings where mask use is recommended to decrease transmission, such as in private gatherings or while inside their homes with a known COVID-19 household contact. For these and other reasons, high reported rates of mask use despite a burgeoning pandemic in the U.S. do not suggest that masks do not work to decrease transmission. Rather, those who use the Delphi data must be aware of its limitations, potential biases and of what it does and does not show, and mask use is one part of a comprehensive response to the virus.
In summary, large-scale self-report surveys can be valuable to public health practice. During the COVID-19 pandemic, mobile phone applications and web-based tools that facilitate collection of self-reported data on a large scale have been deployed by several research groups. There are potential limitations to data gathered this way, and it is important that the public, researchers and public health practitioners are aware of these limitations when analyzing data and interpreting and using results. However, these surveys may yield the best answers to some critical questions about the public health response to the pandemic. Data are centrally collected and results may be rapidly redeployed to inform the public of urgent health information and to guide public health interventions. This may be particularly advantageous during a widespread health event, when collecting data across a large geographic area and when comparing trends between areas and across time may be critical to guide the response. It may be advantageous to disseminate surveys via the internet when people are advised to shelter in place, reduce contacts and maintain physical distance. The low cost of such surveys is an important additional benefit. There is also tremendous power in the scale of the data that may be collected. And critically, during a long-lasting health threat, internet-based data collection platforms allow trends to be monitored consistently over time. For all of these reasons, data from large-scale online surveys have been notable contributors to public health research during the COVID-19 pandemic. Data may also be invaluable to the public health response. For example, a question about willingness to receive a COVID-19 vaccine was recently added to the Delphi survey.
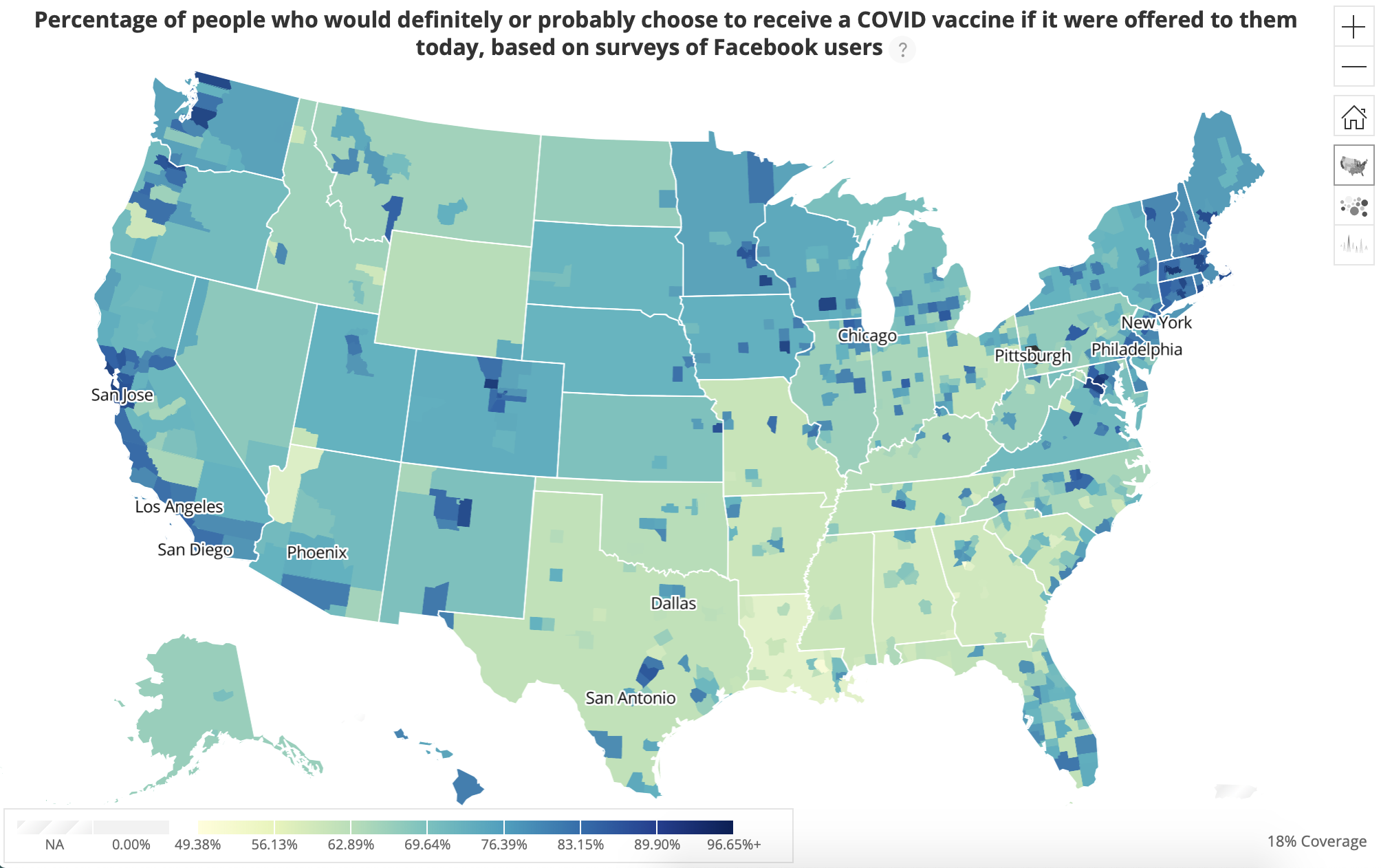
These data on vaccine acceptance are geographically granular to the county level. Collection of these data began on Jan. 1; by Jan. 4, more than 250,000 people were contributing responses to this question each day. Of approximately 275,000 responses gathered on Jan. 13, 73% of people said they would probably or definitely get a COVID-19 vaccine if it was offered to them, but there were highly variable acceptance rates among counties. This adds to what is already known about vaccine hesitancy in the U.S. from other surveys. Although the Delphi data do not currently reveal reasons for vaccine hesitancy, the surveys are likely the most up-to-date, robust data on COVID-19 vaccine acceptance available. Thus, they may serve to inform efforts to improve acceptance of COVID-19 vaccines, a critical tool in the fight to control the pandemic.
Weekly Research Highlights
6-Month Consequences of COVID-19 in Patients Discharged from Hospital: a Cohort Study
(The Lancet, Jan. 8, 2020)
- The study included 1,733 patients who were hospitalized between January and May in Jin-Yin Tan Hospital in Wuhan. 736 patients were excluded, most because it was not feasible to have them come for an in-person interview. The average age was 57, and 52% were men.
- In addition to self-reported symptoms, the researchers assessed lung function and conducted chest CTs in a subset of participants. 23% of those with less severe COVID-19 had impairment in lung diffusion compared to 56% of those who required ventilation or a high flow nasal cannula. There were few major differences in CT results by severity of disease. Kidney function also appears to have declined in 35% of participants compared to during their hospital stay.
- Overall, women were more likely to have residuals symptoms than men.
- Limitations: The study likely underestimates the proportion of patients with long-term effects of COVID-19 as people who could not attend an in person interview were excluded. This included some of the people who had been most seriously ill with COVID-19 (nursing home residents, people readmitted to hospital, those who died during follow-up, etc.). Furthermore, the study did not evaluate people who had not been sick enough to require hospitalization, hence the prevalence of long-term symptoms in this group cannot be addressed from this study.
Time from Start of Quarantine to SARS-CoV-2 Positive Test Among Quarantined College and University Athletes — 17 States, June–October 2020
(MMWR, Jan. 8, 2020)
- A total of 1,830 athletes from 24 colleges and universities were included in the main analysis. 25% (458) received a positive test result, of whom 137 (30%) were asymptomatic. Another 65 were symptomatic but had a negative test result. Social gathering (41%) and roommates (32%) were the most commonly reported exposures rather than athletic activities (13%).
- In a time-to-event analysis including 620 athletes who tested positive for COVID-19 (includes an additional 162 athletes from three schools that only provided information on people with positive tests), the following were found:
- Quarantine started on average 1.1 days after exposure
- The median time to a positive test result from the start of quarantine was two days; the median was 3.8 days
- Test positivity rates declined over time, from 20%–25% to close to 5% by day 14.
- Limitations: The major limitation is that the time-to-event analysis is subject to potential biases in opposite directions.
- First, the analysis relies on tests as they happened to be administered, potentially leading to an overestimate of the time to a first positive test. For instance, 26 of the 29 people who tested positive on days 11-14 of quarantine had not been previously tested.
- In the opposite direction, the date of exposure was often not known. The time-to-event estimates were calculated from the day that quarantine began, potentially leading to an underestimate of length of time to a positive test.
Suggested citation: Cash-Goldwasser S, Kardooni S, Jones SA, Cobb L, Bochner A, Bradford E and Shahpar C. In-Depth COVID-19 Science Review January 9 – 15, 2020. Resolve to Save Lives. 2021 January 20. Available from https://preventepidemics.org/covid19/science/review/